The Power of Machine Learning in Robotic Intelligence
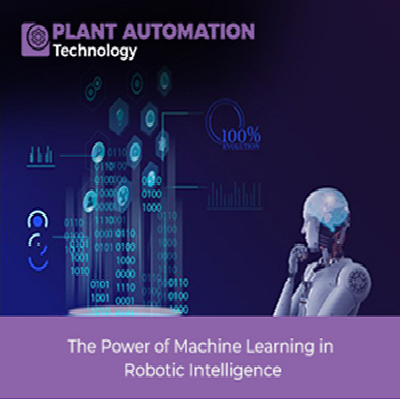
Introduction
Robotic intelligence has undergone significant transformations in recent years, due to machine learning techniques. As artificial intelligence (AI) continues to evolve, machine learning plays a crucial role in propelling robotics to new heights of efficiency, adaptability, and problem-solving capabilities. In this article, we will explore the pivotal role of machine learning in advancing robotic intelligence. We will also explore the profound impact it has on various industries.
Robotic Intelligence through Machine Learning
Training Robots with Machine Learning Algorithms
Machine learning algorithms are crucial in the development of robot intelligence. Robots learn from prior experiences via supervised learning, unsupervised learning, and reinforcement learning, perfecting their decision-making abilities and performing tasks with exceptional precision and accuracy. These developments pave the path for extremely capable and skilled robotic systems.
Supervised learning involves training robots using labeled data, where they learn to make predictions and decisions based on well-defined input-output pairs. In industrial settings, robots trained through supervised learning can identify defects in manufacturing processes with high accuracy, leading to improved product quality and reduced waste.
Unsupervised learning, on the other hand, allows robots to learn from unlabeled data, discovering patterns and relationships on their own. This is particularly valuable in applications like anomaly detection, where robots can identify unusual events or behaviors in complex systems, enhancing safety and predictive maintenance in critical infrastructures.
Reinforcement learning allows robots to learn by trial and error, with feedback in the form of incentives or penalties. This method allows robots to optimize their behavior over time, making them highly adaptable and capable of dealing with changing surroundings. Reinforcement learning, for example, is used in autonomous vehicles to manage complicated traffic scenes, boosting road safety.
Enhancing Perception and Sensing
With advanced machine learning algorithms, robots elevate their perception and sensing capabilities to new heights. Computer vision techniques and sensor fusion enable robots to process visual and sensory data, empowering them to recognize objects, environments, and obstacles in real time. This heightened awareness fosters safer interactions between robots and their surroundings, driving significant progress in diverse industries.
In healthcare, robots equipped with sophisticated computer vision algorithms can assist surgeons during delicate procedures, enhancing precision and reducing surgical errors. Furthermore, robots integrated with sensor fusion technology can navigate hazardous environments, such as disaster-stricken areas, assisting in search and rescue operations and minimizing human risk.
Autonomous Navigation and Mapping
Machine learning revolutionizes robotic navigation, enabling robots to maneuver autonomously through complex environments. Algorithms like simultaneous localization and mapping (SLAM) facilitate real-time mapping of surroundings, while reinforcement learning assists robots in making dynamic decisions during navigation. This autonomy opens up exciting applications in autonomous vehicles, drones, and exploration missions.
Autonomous delivery drones, empowered by SLAM algorithms, can efficiently navigate urban landscapes, delivering packages to customers with unprecedented speed and accuracy. In space exploration, robotic rovers equipped with machine learning capabilities can autonomously explore uncharted terrains on distant planets, expanding our knowledge of the universe.
Human-Robot Interaction
Machine learning enhances the way robots interact with humans. Natural Language Processing (NLP) allows robots to understand and interpret spoken language, promoting seamless communication. Additionally, sentiment analysis empowers robots to gauge human emotions, making interactions more empathetic and personalized. This breakthrough fosters collaborative work environments and enriches user experiences.
In customer service, AI-powered robots with NLP capabilities can provide personalized support, addressing customer queries and resolving issues efficiently. Moreover, in healthcare settings, robots with sentiment analysis skills can offer emotional support to patients, helping improve their overall well-being during their stay in hospitals or care facilities.
Predictive Maintenance and Fault Detection
Machine learning algorithms empower robots to predict maintenance needs and detect faults in their systems. By analyzing sensor data and historical performance, robots identify potential issues before they escalate into critical failures. This predictive maintenance approach enhances the reliability and longevity of robotic systems, minimizing downtime and maintenance costs.
In the manufacturing industry, robots with predictive maintenance capabilities can proactively identify equipment failures, optimize production schedules, and reduce unplanned downtime. Similarly, in smart cities, robots equipped with fault detection algorithms can ensure the timely maintenance of critical infrastructure, enhancing overall efficiency and resource management.
Robotic Learning from Demonstration (LfD)
Machine learning's LfD paradigm enables robots to learn complex tasks by observing and imitating human demonstrations. This approach expedites the deployment of robots in various industries, as they can quickly acquire new skills without explicit programming. This flexibility empowers robots to adapt seamlessly to ever-changing tasks and environments, revolutionizing automation.
In manufacturing, robots equipped with LfD capabilities can be easily reprogrammed to handle new assembly processes, improving production line flexibility and reducing the need for specialized training. Additionally, in education, robots trained through LfD can assist students in learning complex subjects, providing personalized tutoring and support.
Real-time Adaptability and Continuous Improvement
One of the most significant advantages of integrating machine learning into robotic intelligence is the ability to continuously improve and adapt in real time. As robots interact with their environments and complete tasks, they generate valuable data that can be fed back into the learning process. This feedback loop allows robots to refine their decision-making algorithms and fine-tune their actions, leading to constant improvement and increased efficiency over time. As a result, robots become more reliable and effective at handling complex tasks, making them indispensable assets in industries that demand precision and productivity.
Robotic Swarms and Collective Intelligence
Machine learning also facilitates the coordination and collaboration of multiple robots, leading to robotic swarms with collective intelligence. These swarms can work together to achieve common goals, exhibit robustness against individual failures, and adapt to dynamic environments seamlessly. Swarm robotics finds applications in search and rescue operations, environmental monitoring, and even extraterrestrial terrain exploration. By leveraging machine learning to orchestrate these swarms, we unlock the potential for scalable and highly efficient solutions to complex challenges.
Ethical Considerations and Safety Measures
As robotic intelligence advances, ethical concerns and safety precautions become increasingly significant. Machine learning algorithms must be built with robust safety safeguards to avoid unforeseen consequences or dangerous behaviors. Furthermore, robotic intelligence ethics must be rigorously monitored and regulated. This is to guarantee that these technologies are used appropriately and for humanity. Balancing scientific advancement with appropriate guidelines will be critical to securing robotic intelligence's widespread acceptance and integration in our societies.
The Future of Robotics and Machine Learning Integration
Looking ahead, robotics and machine learning will continue to shape the future across sectors. The quest for artificial general intelligence (AGI) will further push robotic capabilities, allowing them to reason, plan, and solve problems in more complex and unstructured environments. With advancements in neural network architectures and computational power, robots will become even more proficient at handling large-scale data and making intricate decisions, expanding their potential applications in fields like finance, healthcare, and scientific research.
Conclusion
Machine learning and robotics have transformed artificial intelligence, driving unprecedented robotic intelligence progress across multiple industries. From enhancing perception and navigation to promoting human-robot interaction, predicting maintenance needs, and enabling automated swarms, the possibilities seem limitless. Nevertheless, as we embrace these cutting-edge technologies, it is essential to remain vigilant about ethical considerations and safety measures, ensuring that the future of robotic intelligence serves humanity's best interests. With continued research and responsible implementation, the future holds immense potential for machines that are not only intelligent but also empathetic collaborators in our daily lives.